Language-Grounded Indoor 3D Semantic Segmentation in the Wild
1Technical University of Munich 2NVIDIA
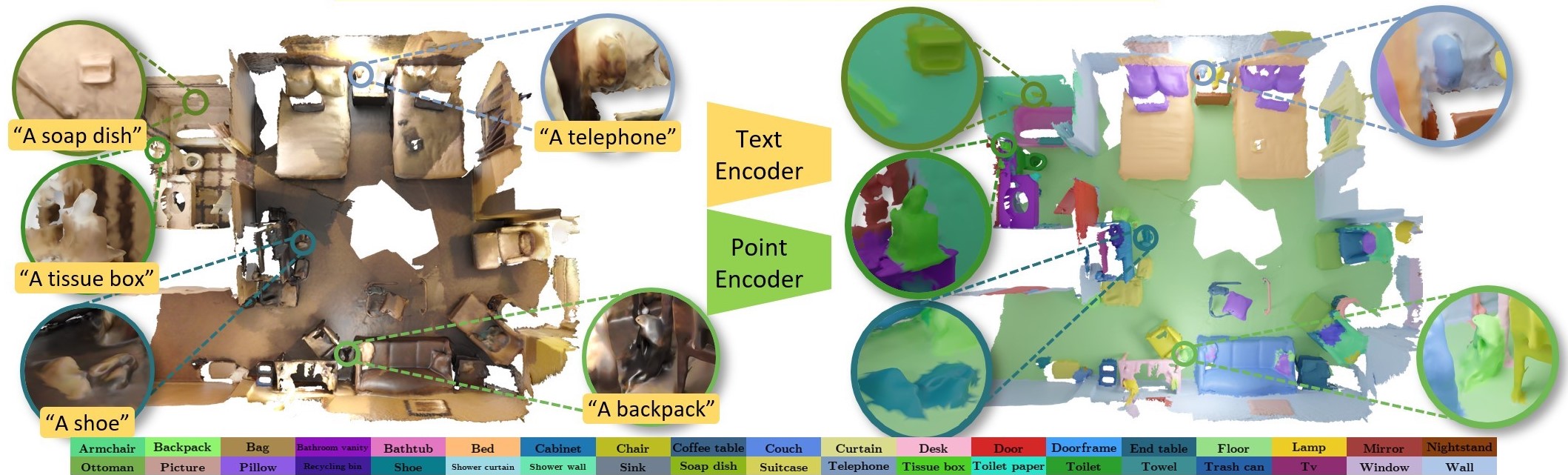
We present the ScanNet200 benchmark, which studies 200-class 3D semantic segmentation - an order of magnitude more class categories than previous 3D scene understanding benchmarks. To address this challenging 3D segmentation task, we propose to guide 3D feature learning by anchoring it to the richly-structured text embedding space of CLIP for the semantic class labels. This results in improved 3D semantic segmentation across the large set of class categories.
Video
ScanNet200 Benchmark
The ScanNet Benchmark has provided an active online benchmark evaluation for 3D semantic segmentation, but only considers 20 class categories, which is insufficient to capture the diversity of many real-world environments. We thus present the ScanNet200 Benchmark Benchmark for 3D semantic segmentation with 200 class categories, an order of magnitude more than previous. In order to better understand performance under the natural class imbalance of the ScanNet20benchmark, we further split the 200 categories into sets0 of 66, 68 and 66 categories, based on the frequency of number of labeled surface points in the train set: head, common and tail respectively. A comparison of the class distibution of surface annotated points in the original and our propoed benchmark can be seen in the figure above.
Language-Grounded Learning
During pre-training, we guide 3D feature learning by mapping learned features to text encoded anchors of the corresponding semantic labels, constructed by a constrastive loss between text and 3D. This establishes a more robust 3D feature representation space guided by the rich structure of the text embeddings. We leverage a pre-trained CLIP to map semantic labels to text features. Note that our approach is agnostic to the specific language model used, but we found CLIP's multi-modal training is well-suited to our language-3D pre-training. In this stage, the the text encoder is pre-trained and fixed, and takes the ScanNet200 target semantic labels in their text form and projects them in the joint representation space. For 3D feature extraction, we employ a state-of-the-art sparse 3D convolutional U-Net implemented with the MinkowskiEngine framework. We then train the 3D feature encoder to map to the well-structured space of the text model by formulating a contrastive objective to bring together the different data modalities.
Segmentation Results
Input Meshes | Our Predictions | ScanNet200 annotations |
| | |
| | |
| | |
BibTeX
If you find our project useful, please consider citing us:
@inproceedings{rozenberszki2022language,
title={Language-Grounded Indoor 3D Semantic Segmentation in the Wild},
author={Rozenberszki, David and Litany, Or and Dai, Angela},
booktitle = {Proceedings of the European Conference on Computer Vision ({ECCV})},
year={2022}
}